Degeneracy and Jefferson and a Moose
17 Mar 2021
“The Europeans who pass into America degenerate, as do the animals; a proof that the climate is unfavourable to the improvement of either man or animal… This degradation of humanity must be imputed to the vitiated qualities of the air stagnating in their immense forests, and corrupted by the noxious vapours from standing waters and uncultivated grounds.”
Apparently around the Revolutionary period in America, one of the most popular theories in Europe about human and animal origins was degeneration theory. Basically the idea that humans originated in the same place, but that the reason they were different in different places now is that some ended up living in different places with unfavorable climates, leading them to “devolve” and decline from their original complexity in many places. Of course, being a European theory the idea was that Europeans had not degenerated much or at all.
Obviously this was a theory motivated by racism, used to explain the “inferiority” of other races and peoples. But a funny aspect of it is the controversy it caused with American colonists. The dopes pushing the theory implied that the American colonists had become inferior too by being exposed to the climate of North America. The idea was that the North American climate was bad for living creatures, resulting in toxic plants, which infected people and animals and made them smaller and feebler of mind and body. Some of the founding fathers got offended by Europeans calling them inferior and spent time criticizing the theory. Thomas Jefferson even had a stuffed moose sent to one of the originators of the theory to say “hey idiot, look at how massive this thing is, call my moose small and feeble, I dare you.”
The quote at the start of this post and all of the hilariously incorrect quotes below come from a Dutch philosopher named Cornelius de Pauw who was one of the proponents of degeneration theory. The source is from a translation of his book at the link, you can look at it for free. Just prepare yourself for some horrifying racism and stupidity: https://www.google.com/books/edition/Selections_from_Les_Recherches_philosoph/4WRZAAAAcAAJ?hl=en&gbpv=1
“It was observed, at the discovery of the new world, that there was no such thing as a large quadruped to be found between the Tropics; there were neither horses, asses, oxen, camels, dromedaries, nor elephants; all which, except the last, have from time immemorial been so effectually tamed to the services of man in our hemisphere.”
“The Puma, or lion of America, has no mane; nor is it to be compared for size, force, or courage, with the lion of Africa. The same may be affirmed of their Jaguar, which has been honoured with the name of tiger; as to the Couguar, or poltroon tiger, as it is called, it seems to be peculiar to this country.”
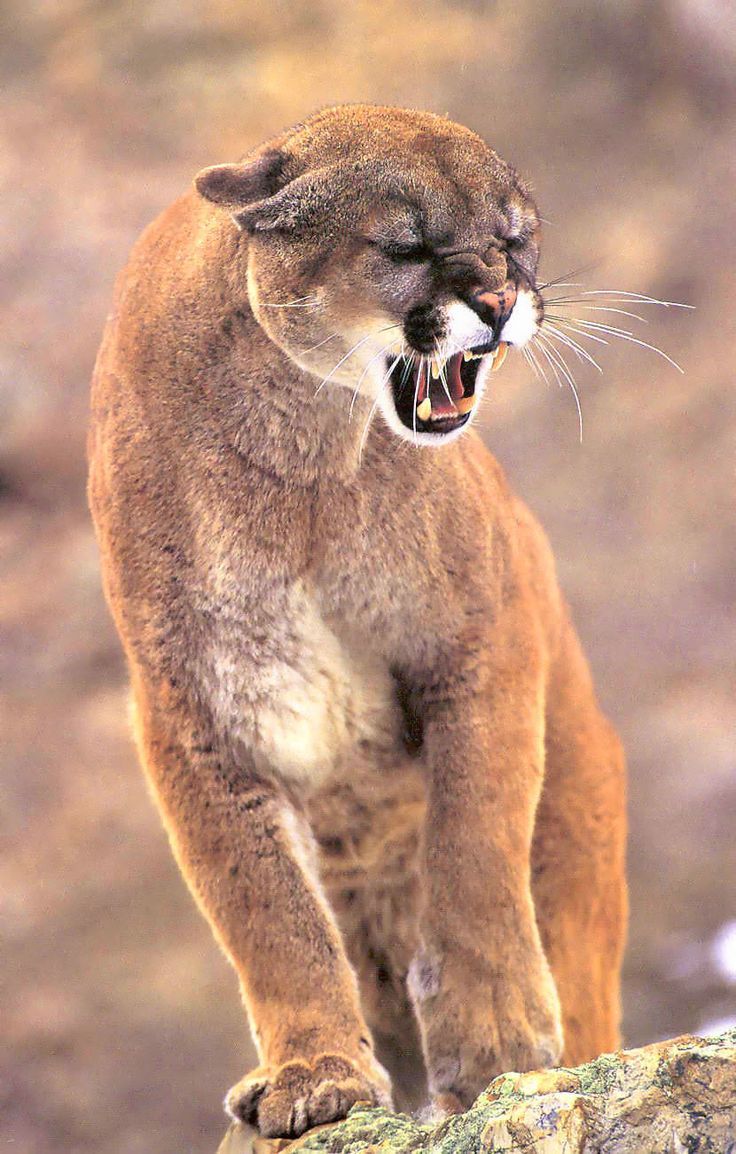
“It is certain that many animals have been found in the new world, which have not their familiars in the old; from which we may infer, that the two continents were never united under the equator; for if the sea between Guinea and the Brazil had ever been terra firma, the animals of the Torrid Zone of the two hemispheres would be found on each continent: whence it follows, that each climate hath received from Nature its appropriated species.”
“To the malignity of the air of America must be imputed the prodigious propagation of insects, venomous serpents, and infected vegetations, which so unhappily distinguish the hemisphere. The same ill qualities of the air which are favourable to these noxious productions, are probably the true origin of the degeneracy in men and animals; as the same corrupt juices which infect the vegetable nature, must taint the blood, and subdue the powers of the animal.”
A wikipedia article on degeneration theory: https://en.wikipedia.org/wiki/Degeneration_theory
More info on the story of how Thomas Jefferson sent a dead moose to Paris: https://www.monticello.org/site/research-and-collections/american-moose